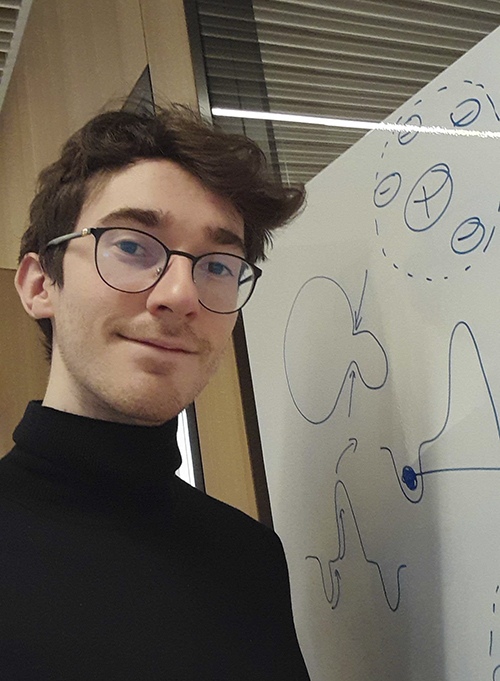
Dr. Paul Robin's Ionic Computing Revolution: Artificial Neurons Shaping the New Era of Future Electronic Memory
We recently had the honor to interview Dr. Paul Robin, who received mentorship from Lydéric Bocquet at ENS and is now affiliated with the Institute of Science and Technology Austria. A pioneering figure in the realm of artificial neurons, Dr. Robin and his team have conducted research that was featured in Science magazine on August 6, 2021. Their work focuses on brain-inspired electronics and was a joint effort with scientists from CNRS and École Normale Supérieure – PSL. The research emphasizes the creation of artificial neurons utilizing ions and investigates the use of graphene nanoslits with a singular water layer for neuron-like signal transmission. This study underscores the significance of nanofluidics in the development of energy-efficient electronics, aiming to replicate the human brain’s capability to function on minimal energy and its potential applications in electronic memory and artificial intelligence. During our discussion with Dr. Robin, we delved deeper into his innovative research, shedding light on the significant impacts of his work on artificial neurons and brain-inspired electronics.
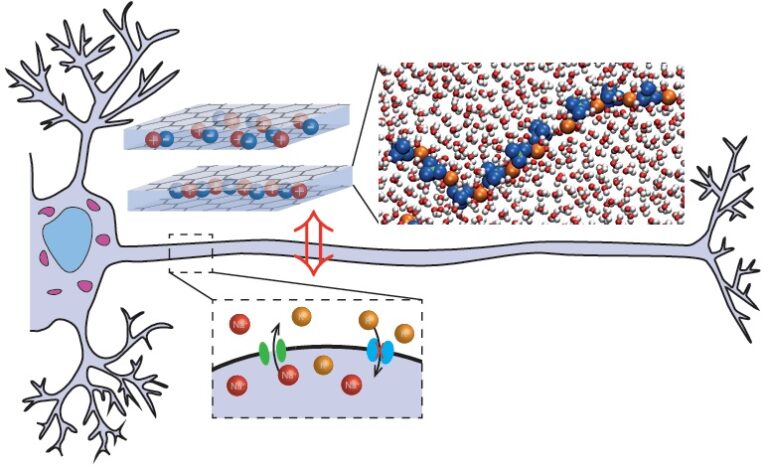
What inspired your team to explore the development of artificial neurons using ions instead of electrons?
We had this idea for quite some time but my research was not at all focused on that. However, by modeling the properties of atomically-small pores made of graphene, we discovered that under certain conditions they could behave like cellular pores found in neurons.
In your groundbreaking paper published in Science, you discuss the use of graphene nanoslits to mimic the function of neurons. Could you explain this technology to our readers in layman’s terms?
Neurons communicate with one another with action potentials, short pulses of voltage. They generate these through the activation of very small pores on the membrane of neurons. These pores can control the flows of ions by opening and closing at certain frequencies, creating bursts of voltage. These graphene slits display a similar phenomenon, albeit with a different underlying mechanism: ions inside these slits tend to assemble into ion pairs, which block electrical current. These pairs have a certain lifetime, after which they will tend to break and to allow electrical conduction. This also results in voltage bursts, just like in neurons.
Can you elaborate on the significance of energy efficiency in artificial neurons and how it compares to the human brain?
The field is currently in its infancy so we generally do not focus on energy efficiency: creating an artificial neuron is already hard enough! However we do know that there is a good margin for improvement in terms of energy efficiency: our brain functions with a power of 20W (equivalent to a small lightbulb), which is much less than our modern computers.
How does nanofluidics contribute to the study of artificial neurons, and what potential does it hold for future developments?
Nanofluidics, which is the science of measuring and controlling flows at the molecular scale, offers a good deal of potential (and, in some cases, already developed) applications: water desalination, energy harvesting, filtration, etc. At the moment, artificial neurons are little more than a curiosity, but they show how we can artificially control flows of ions at the molecular scale. In and of itself, that is already a great achievement. It could help understanding certain processes in biology or developing new types of membranes for the applications listed above. Bio-inspired computing is a long-term horizon: we are still not quite there, but we have faith that great progress could be achieved in the years to come!
Your study mentions the ‘memristor effect’ in relation to artificial neurons. Could you explain what this effect is and how it contributes to the functionality of these neurons?
A memristor is an electrical component whose conductivity depends on its history: it is an elementary component to make computing devices ‘learn’ things from their environment. People have realized that the same equations that describe this ‘memory’ effect can be used to describe how cellular pores open and close to the passage of ions in neurons. The memristor effect is what allows neurons to emit action potentials, which they use to communicate.
The practical application of your research is fascinating. How close are we to seeing these artificial neurons implement simple learning algorithms, and what are the challenges you face in achieving this?
There are several steps that need to be made.
The first one is to observe this memory effect in experiments, as my paper is ‘only’ a theoretical prediction. This observation has now been made, in collaboration with colleagues doing experiments (see eg. my recent publication Robin, Emmerich, Ismail et al., Science, 2023).
Another one would be to be able to connect a few of these systems together to implement basic operations. Some preliminary results exist for this, see the works of my colleagues Emmerich, Ronceray et al. (it will be published in the coming weeks). They managed to connect two systems together to implement logical operations.
The last step would be to connect many of these systems – say dozens or hundreds – to create biomimetic neuron networks. This is currently extremely challenging and no one knows how to do that. However, research progresses in a very much non-linear way, so who knows what the future holds in store!
Given the high energy consumption of current AI technologies, how will the development of ion-based neurons change the landscape?
In the short run it will not change much as we are nowhere near the level of complexity of modern computers. My best guess is that in the long run we may develop hybrid technologies where electronics (the flows of electrons) controls iontronics (the flows of ions) and vice-versa.
What are the next experimental milestones your team aims to achieve, and what will be the focus of your upcoming research?
I have since then left that team as I am now working in Vienna. However, colleagues have continued these efforts ever since. My theoretical predictions were validated, and now the next big goal is to find how to connect these fluidic systems to make them interact.
What are the steps involved in moving from the theoretical model you’ve developed to a practical, working system?
To do that you need to extract the underlying principles behind the theoretical results. There are often many differences between models and actual experimental systems, so you need a good grasp of what is crucial to obtain the desired effect and what is not. Then, you need to test out different setups and conditions to find what are the best ones for the experiments.
How do you see your work impacting broader technological advancements and society as a whole, particularly in terms of sustainability and energy consumption?
I believe that reducing the energy consumption of computing systems by using nanofluidic devices is not necessarily the biggest impact of my research, as this technology is far from mature. However, it may help understanding how Nature manages to carry out complex operations at low energy cost; answering this question on a fundamental level would have far deeper consequences.
With the advancement of brain-like electronics, what ethical considerations do you believe should be addressed in the research community?
I believe that the general public has a very inaccurate perception of what “brain-like computing” or ‘machine learning’ actually means. We are not creating electronic devices that could outsmart the brain anytime soon. And even if we were, ethical problems concern rather the use of these technologies than the technologies themselves. For example, machine learning is, on a fundamental level, little more than a very big statistical analysis (looking at many examples of a problem to predict ways of solving it in future cases). The big problem is not the ‘algorithm’ part, which is in truth rather simple, but how people acquire data to make these algorithms work: currently they (eg. ChatGPT) simply steal data on the internet without the owners’ consent.